With AI models clobbering every benchmark, it's time for human evaluation


Artificial intelligence has traditionally advanced through automatic accuracy tests in tasks meant to approximate human knowledge.
Carefully crafted benchmark tests such as The General Language Understanding Evaluation benchmark (GLUE), the Massive Multitask Language Understanding data set (MMLU), and "Humanity's Last Exam," have used large arrays of questions to score how well a large language model knows about a lot of things.
However, those tests are increasingly unsatisfactory as a measure of the value of the generative AI programs. Something else is needed, and it just might be a more human assessment of AI output.
Also: AI isn't hitting a wall, it's just getting too smart for benchmarks, says Anthropic
That view has been floating around in the industry for some time now. "We've saturated the benchmarks," said Michael Gerstenhaber, head of API technologies at Anthropic, which makes the Claude family of LLMs, during a Bloomberg Conference on AI in November.
The need for humans to be "in the loop" when assessing AI models is appearing in the literature, too.
In a paper published this week in The New England Journal of Medicine by scholars at multiple institutions, including Boston's Beth Israel Deaconess Medical Center, lead author Adam Rodman and collaborators argue that "When it comes to benchmarks, humans are the only way."
The traditional benchmarks in the field of medical AI, such as MedQA created at MIT, "have become saturated," they write, meaning that AI models easily ace such exams but are not plugged into what really matters in clinical practice. "Our own work shows how rapidly difficult benchmarks are falling to reasoning systems like OpenAI o1," they write.
Rodman and team argue for adapting classical methods by which human physicians are trained, such as role-playing with humans. "Human-computer interaction studies are far slower than even human-adjudicated benchmark evaluations, but as the systems grow more powerful, they will become even more essential," they write.
Also: 'Humanity's Last Exam' benchmark is stumping top AI models - can you do any better?
Human oversight of AI development has been a staple of progress in Gen AI. The development of ChatGPT in 2022 made extensive use of "reinforcement learning by human feedback." That approach performs many rounds of having humans grade the output of AI models to shape that output toward a desired goal.
Now, however, ChatGPT creator OpenAI and other developers of so-called frontier models are involving humans in rating and ranking their work.
In unveiling its open-source Gemma 3 this month, Google emphasized not automated benchmark scores but ratings by human evaluators to make the case for the model's superiority.
Google even couched Gemma 3 in the same terms as top athletes, using so-called ELO scores for overall ability.
Also: Google claims Gemma 3 reaches 98% of DeepSeek's accuracy - using only one GPU
Similarly, when OpenAI unveiled its latest top-end model, GPT-4.5, in February, it emphasized not only results on automated benchmarks such as SimpleQA, but also how human reviewers felt about the model's output.
"Human preference measures," says OpenAI, are a way to gauge "the percentage of queries where testers preferred GPT‑4.5 over GPT‑4o." The company claims that GPT-4.5 has a greater "emotional quotient" as a result, though it didn't specify in what way.
Even as new benchmarks are crafted to replace the benchmarks that have supposedly been saturated, benchmark designers appear to be incorporating human participation as a central element.
In December, OpenAI's GPT-o3 "mini" became the first large language model to ever beat a human score on a test of abstract reasoning called the Abstraction and Reasoning Corpus for Artificial General Intelligence (ARC-AGI).
This week, François Chollet, inventor of ARC-AGI and a scientist in Google's AI unit, unveiled a new, more challenging version, ARC-AGI 2. While the original version was scored for human ability by testing human Amazon Mechanical Turk workers, Chollet, this time around, had a more vivid human participation.
Also: Google releases 'most intelligent' experimental Gemini 2.5 Pro - here's how to try it
"To ensure calibration of human-facing difficulty, we conducted a live study in San Diego in early 2025 involving over 400 members of the general public," writes Chollet in his blog post. "Participants were tested on ARC-AGI-2 candidate tasks, allowing us to identify which problems could be consistently solved by at least two individuals within two or fewer attempts. This first-party data provides a solid benchmark for human performance and will be published alongside the ARC-AGI-2 paper."
It's a little bit like a mash-up of automated benchmarking with the playful flash mobs of performance art from a few years back.
That kind of merging of AI model development with human participation suggests there's lots of room to expand AI model training, development, engineering, and testing with greater and greater concentrated human involvement in the loop.
Even Chollet cannot say at this point whether all that will lead to artificial general intelligence.
Want more stories about AI? Sign up for Innovation, our weekly newsletter.
What's Your Reaction?
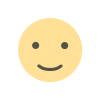
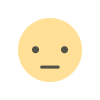
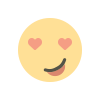
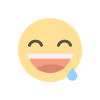
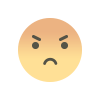
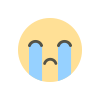
