Researchers get spiking neural behavior out of a pair of transistors
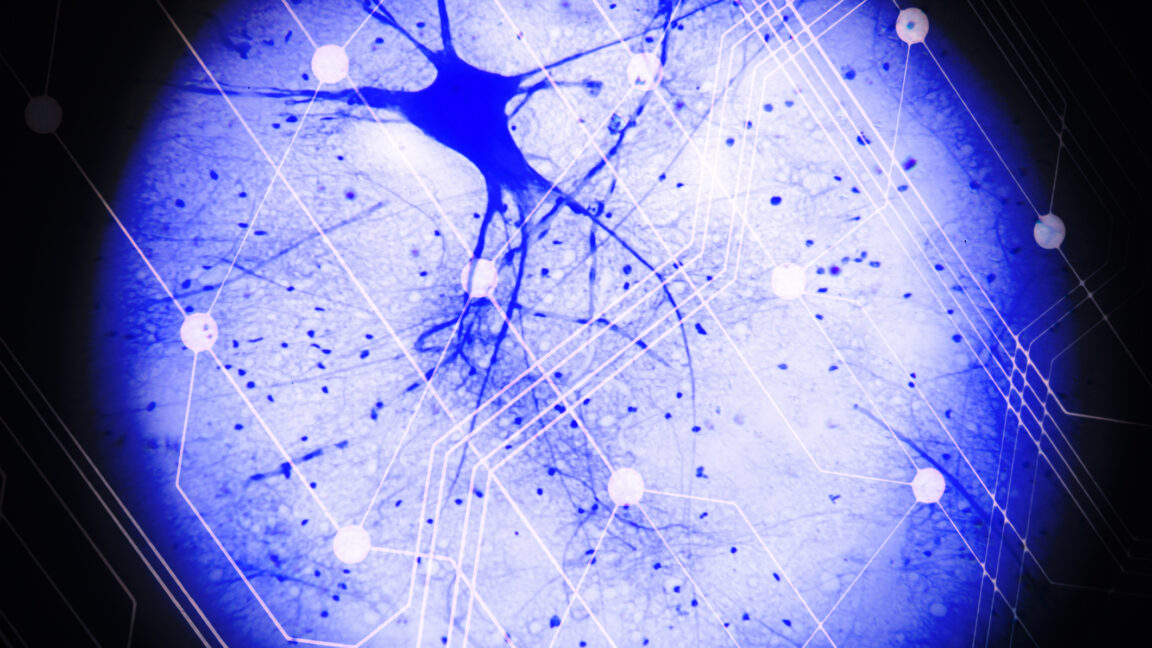
The team found that, when set up to operate on the verge of punch-through mode, it was possible to use the gate voltage to control the charge build-up in the silicon, either shutting the device down or enabling the spikes of activity that mimic neurons. Adjustments to this voltage could allow different frequencies of spiking. Those adjustments could be made using spikes as well, essentially allowing spiking activity to adjust the weights of different inputs.
With the basic concept working, the team figured out how to operate the hardware in two modes. In one of them, it acts like an artificial synapse, capable of being set into any of six (and potentially more) weights, meaning the potency of the signals it passes on to the artificial neurons in the next layer of a neural network. These weights are a key feature of neural networks like large language models.
But when combined with a second transistor to help modulate its behavior, it was possible to have the transistor act like a neuron, integrating inputs in a way that influenced the frequency of the spikes it sends on to other artificial neurons. The spiking frequency could range in intensity by as much as a factor of 1,000. And the behavior was stable for over 10 million clock cycles.
All of this simply required standard transistors made with CMOS processes, so this is something that could potentially be put into practice fairly quickly.
Pros and cons
So what advantages does this have? It only requires two transistors, meaning it's possible to put a lot of these devices on a single chip. "From the synaptic perspective," the researchers argue, "a single device could, in principle, replace static random access memory (a volatile memory cell comprising at least six transistors) in binarized weight neural networks, or embedded Flash in multilevel synaptic arrays, with the immediate advantage of a significant area and cost reduction per bit."
What's Your Reaction?
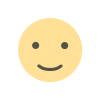
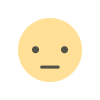
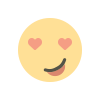
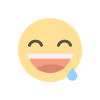
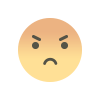
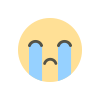
